Frequently Asked Questions
Cloud Optical Properties
Q: How do I assess the quality of the cloud optical and cloud microphysical retrievals?
A: In Collection 5, a Confidence QA bit flag (values from 0 for no confidence to 3 for high confidence) within the Quality_Assurance_1km SDS was assigned to each retrieval that, in addition to the pixel-level retrieval uncertainty, provided some measure of retrieval quality. In Collection 6, however, the Confidence QA is set to 3 (i.e., high confidence) for all successful retrievals such that it is no longer useful for quality assessment. Nevertheless, sufficient information is provided in accompanying SDSs for users to infer retrieval quality. Because large pixel-level retrieval uncertainty implies the observed reflectances lie in a portion of the LUT solution space that is less sensitive to the retrieved quantity, users are advised to determine retrieval quality in part via retrieval uncertainty. Users are also encouraged to look at the new Cloud_Mask_SPI SDS that provides the sub-pixel heterogeneity index. See Section 2.10.13 of the [MOD06 User Guide]. Large sub-pixel heterogeneity has been shown to be associated with retrieval biases [Zhang et al., 2012] and increased retrieval failure rates [Cho et al., 2015]. Likewise, users can also query the Multi Layer Cloud Flag that is included in the Quality_Assurance_1km SDS. See Section 2.10.1 of the [MOD06 User Guide] for details and recommended usage, as multi-layer cloud scenes are problematic for retrievals such as MOD06 that assume a single cloud phase. Finally, in some instances the cloud top retrievals may fail (e.g., due to known saturation issues with the 14µm CO2 slicing channel), in which case the MOD06 optical and microphysical retrievals default to the surface temperature and pressure for the cloud top assumption and atmospheric corrections, thus yielding suspect retrievals. Users are advised that MOD06 optical and microphysical retrievals that have corresponding 1km cloud top temperature or pressure retrievals set to fill values should be discarded [Platnick, et al., 2017].
Q: There are three spectral cloud effective radius (CER) retrievals. Which should I use, and how do I interpret their differences?
A: It depends on your particular application. While the three spectral channels have been shown to have different penetration depths within a plane-parallel, vertically inhomogeneous cloud [Platnick, 2000], users should nevertheless be cautious drawing conclusions from CER retrieval differences, e.g., inferring vertical cloud droplet size distributions. Errors in atmospheric corrections, the 3.7µm emission correction, etc., may yield artifacts in the spectral CER differences. In addition, 1.6µm CER retrievals from Aqua MODIS require greater scrutiny due to known non-functioning detectors and potential unknown issues with the remaining functional detectors. See Section 2.5 of the [MOD06 Users Guide].
Q: Why are there multiple spectral cloud optical thickness (COT) retrievals?
A: To provide the three spectral cloud effective radius (CER) retrievals, the full retrieval process is run for each dual spectral channel combination, and therefore yields three independent COT retrievals. In most cases these three COT retrievals will be nearly identical since each is derived from the same VNSWIR channel reflectance. However, in non-orthogonal regions of the pre-computed look-up table solution space where the SWIR or MWIR reflectance can influence the COT retrieval result, for instance at small COT, the three COT retrievals may differ. Furthermore, because the spectral CER retrievals are known to fail at different rates and under different conditions [Cho et al., 2015], the cloudy pixel population having successful COT and CER retrievals will be different for each spectral combination. It was therefore decided to report each COT retrieval independently, instead of providing a single combined COT dataset, such that each spectral CER retrieval will have a consistent COT retrieval.
Q: Do spectral cloud effective particle radius (CER) retrieval differences provide information about cloud vertical size distribution?
A: While the 1.6, 2.1, and 3.7 µm channels have been shown to have different vertical photon penetration depths within cloud [Platnick, 2000], and thus the respective CER retrievals may be “sampled” from different portions of the cloud, users are cautioned from drawing such inferences from the aggregated L3 scalar statistics. Because the spectral COT-CER retrievals are known to fail at different rates and under different circumstances [Cho et al., 2015], the resulting differences in retrieval populations may introduce biases into the aggregated retrievals. Moreover, radiometric issues and/or retrieval errors, i.e., those due to atmospheric correction, emission correction at 3.7µm, etc., may also play a role.
Q: What does “PCL” mean?
A: The modifier PCL stands for “partly cloudy,” and refers to the L2 pixel population determined to be either partially cloud-covered or at cloud edge as determined by the MOD06 Clear Sky Restoral (CSR) algorithm (see Section 2.8 of the MOD06 User Guide). These pixels are expected to deviate from the retrieval assumptions of an overcast homogenous cloudy FOV and 1-dimensional plane-parallel radiative transfer, conditions that have been shown to be associated with retrieval biases [Zhang et al., 2012] and increased retrieval failure rates [Cho et al., 2015]. Previously in C5, this pixel population was “restored to clear sky” and was excluded from the MOD06 retrievals. In C6, however, optical and microphysical property retrievals are now attempted on these pixels, and successful retrievals are reported in L2 and aggregated to L3, though they are reported separately in the PCL SDSs and are segregated from the “overcast” pixel population.
Q: How do I interpret the PCL (partly cloudy) retrievals?
A: The PCL SDSs contain retrievals for those pixels that are identified as partly cloudy or cloud edge by the Clear Sky Restoral (CSR) algorithm. See Section 2.8 of the [MOD06 User Guide]. These pixels are expected to deviate from the retrieval assumptions of an overcast homogenous cloudy FOV and 1-dimensional plane-parallel radiative transfer, conditions that have been shown to be associated with retrieval biases [Zhang et al., 2012] and increased retrieval failure rates [Cho et al., 2015].
Q: What is the Retrieval Failure Metric and how is it useful?
A: The Retrieval_Failure_Metric (RFM) SDSs represent an attempt to provide additional information about COT and CER retrieval failures, specifically the look-up table (LUT) COT and CER values nearest to the observed reflectances (when applicable) and a Cost Metric that provides a measure of the “degree of failure,” i.e., the relative distance of the observed reflectances from the LUT solution space. The RFM COT, CER, and Cost Metric parameters are assigned values such that the user can ascertain how a given spectral retrieval failed. Details of the RFM SDS assignments and interpretation can be found in Section 2.6 of the [MOD06 User Guide].
Q: What if I would prefer to use my own ice particle habit assumptions instead of those used in producing your ice LUTs?
A: For Collection 6, we are for the first time providing our assumed bulk ice and liquid phase single-scattering properties (i.e., extinction efficiency Qe, asymmetry parameter g, and single scatter albedo ω0) within each MOD06 HDF file. See Section 2.10.2 of the [MOD06 User Guide]. These properties can be used to scale the cloud optical retrievals to the radiative model of your choice! For instance, the cloud optical thickness from MOD06 (τ) can be scaled to that of a different ice model (τ*) using similarity rules [van de Hulst, 1974].
Q: What is the definition of a daytime pixel for Cloud Optical Properties?
A: The cloud optical and microphysical property retrievals define daytime pixels as those having solar zenith angle less than 81.36°. Note this definition differs from that of the cloud top property and IR phase retrievals, as well as the MOD35 cloud mask, that define daytime pixels as those having solar zenith angle less than 85°.
Cloud Top Properties
Q. Can you explain how the Cloud Fraction (from Cloud Mask) is computed in the Cloud (06_L2) Product?
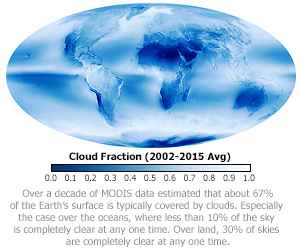
A. The cloud fraction that garners the most interest from MODIS data users is cloud fraction derived directly from the 35_L2 Cloud Mask. (Note the 35_L2 Cloud Mask flags are duplicated in the 06_L2 Cloud product). These Level-2 (L2) and Level-3 (L3) cloud mask cloud fraction SDSs have the prefix
-
Cloud_Fraction
-
Cloud_Fraction_Day
-
Cloud_Fraction_Night
The first parameter listed above contains both day and night retrievals, the second parameter contains daytime only retrievals (solar zenith angle less than or equal to 85°), and the third parameter contains nighttime only retrievals (those that don't meet the daytime criteria).
The L2 cloud fraction from cloud mask, which L3 uses to compute global statistics, is calculated in the cloud top properties algorithm and stored in the 06_L2 HDF file. The L2 cloud mask cloud fraction is derived in L2 by reading the 1×1 km Cloud Mask “Cloudiness Probability Flags” or “Cloudiness Flags” (see Table), which are described on page 27 of the [QA Plan].
L2 QA Flag | Flag Value | Meaning |
---|---|---|
Cloud Mask Status Flag |
0 1 |
Undetermined Determined |
Cloud Mask Cloudiness Flag |
0 1 2 3 |
Confident Cloudy (or Fill if Status Flag = 0) Probably Cloudy Probably Clear Confident Clear |
Table. Two key L2 Cloud Mask Flags used to compute the L2 Cloud Fraction
The Cloud Mask Cloudiness Flag shown in the above table, can have the following settings: 0 = confident cloudy, 1 = probably cloudy, 2 = probably clear, and 3 = confident clear. In the computation of the L2 cloud mask cloud fraction, the first two flags (confident cloudy and probably cloudy) are assigned 100% cloudy and the last two flags (probably clear and confident clear) are assigned 100% clear. Then in each 5×5 km L2 retrieval box, the average L2 cloudiness is computed by computing the fraction of the 1-km pixels that are cloudy divided by the total number of pxels. It should be noted that n the L3 Daily (D3) product, the cloud mask cloud fraction (which have the same SDS prefix's as shown above) is computed by taking an unweighted average of these 5×5 km L2 cloud fractions that fall in each 1°×1° L3 grid box.
Q: What are the differences between the various cloud phase products?
A: There are two cloud phase algorithms included in MOD06. The first is an infrared (IR) based algorithm that is run in parallel with the cloud top (CT) property retrievals. It provides cloud phase at 1km and 5km spatial resolution for both daytime and nighttime, with results reported in the Cloud_Phase_Infrared SDSs. Details of this algorithm can be found in this paper [Baum et al., 2012]. The second algorithm, run as part of the cloud optical property retrieval algorithm, provides the final phase decision for the COT and CER retrievals and derived cloud water paths (CWP). It employs a variety of shortwave-infrared (SWIR) based tests, in addition to information from the CT and IR phase retrievals, thus yielding phase results for daytime only. Results from this algorithm are reported in the Cloud_Phase_Optical_Properties SDS and as a QA bit flag in the Quality_Assurance_1km SDS. Details of this algorithm, including changes for C6, can be found in [Marchant et al., 2016] as well as in Section 2.4 of the [MOD06 User Guide].
Q: Why do cloud top retrievals sometimes have anomalous “boxes” or striping?
A: These features, that usually only appear in the highest altitude cloud top retrievals, are caused by mismatches between the observed infrared (IR) radiances and the 1° resolution NWP model profiles of temperature, moisture, and ozone that are necessary inputs to the CO2 slicing algorithm. Note that no spatial interpolation is performed on the NWP model output, i.e., the profiles are used at their native 1° spatial resolution.
Q: Which cloud top property retrievals should I use. Should I use 1km or should I use 5km?
A: For C6 a number of updates and improvements were made to the cloud top property products (cloud top pressure, height, temperature and cloud effective emissivity) that are documented extensively in [Menzel et al., 2008]. In C5 and earlier versions, these parameters were available only at 5km spatial resolution. In C6, these parameters are now available for the first time at the same 1km resolution as the cloud optical and microphysical properties. Additional information, including comparisons of C6 cloud top heights to those derived from the CALIOP lidar, are available in [Baum et al., 2012]. Users should choose between the 1km and 5km products based on individual needs. For example, the 1km product is best if finer spatial resolution is a paramount concern, and the 5km version is suggested for comparisons with heritage data such as HIRS. The 5km product generally exhibits higher signal to noise characteristics, i.e., more spatially consistent retrievals when clear-sky minus cloudy-sky radiance differences are very small.
Q: Which IR cloud phase retrieval should I use. Should I use 1km or should I use 5km?
A: For C6 a number of updates and improvements were made to the IR cloud phase that are documented extensively in [Baum et al., 2012], and like the cloud top property retrievals, in C6 it is now available for the first time at the same 1km resolution as the cloud optical and microphysical properties. Because the older framework for the 5km IR phase retrieval could not be easily modified without a complete rewrite, among other constraints, algorithm development and evaluation focused instead on the 1km product. However, instead of replacing the existing 5km IR phase product with the new 1km version, the algorithm development team decided to maintain in C6 a version of the 5km product for continuity with C5. Users should be aware that the processing framework changed significantly for the 1km IR phase retrieval, and differences between the 1km and 5km products should therefore be expected. The new 1km IR cloud phase underwent extensive testing and evaluation through comparison with CALIPSO/CALIOP products, and is expected to be of better quality than the heritage 5km products. Furthermore, it is likely that the 1km and 5km products will continue to diverge as further improvements to the 1km products are made over time. Users are thus advised to use the 1km IR phase product both now and in the future.
Q: What is the definition of a daytime pixel for Cloud Top Properties
A: The cloud top property and IR phase retrievals, as well as the MOD35 cloud mask; define daytime pixels as those pixels having solar zenith angle less than 85°. Note this definition differs from that of the cloud optical and microphysical property retrievals that define daytime pixels as those having solar zenith angle less than 81.36°.
Cirrus Detection
Q: How do I interpret the Cirrus_Reflectance and Cirrus_Reflectance_Flag SDSs?
A: While very minor updates were made for C6, the algorithm that produces this dataset is no longer being supported by NASA. Please direct queries to product developer Dr. Bo-Cai Gao at gao@nrl.navy.mil.
Miscellaneous
Q: The MOD06 files only include 5km resolution Latitude and Longitude SDSs. How do I obtain geolocation information for the 1km cloud products?
A: In early collections of MOD06, only the 5km Latitude and Longitude SDSs were included in the files in order to minimize file size. While file size is no longer a concern, the geolocation SDSs in the files remain at 5km resolution for historical reasons. To obtain the 1km geolocation data, users can either interpolate/extrapolate the 5km SDSs to 1km resolution, or download the MOD03 geolocation file that corresponds to the granule(s) of interest.
Q: Many retrieval parameters are stored as integers in the HDF file. How do I convert these to something useful?
A: HDF files are self describing, so local attributes are attached to each and every Scientific Data Set (SDS). These local attributes contain information such as valid_range, scale_factor, add_offset. Nearly all MOD06 retrieval parameters are stored as 16-bit integers to reduce the HDF file size. To convert these back to useful floating point values, one must first read the scale_factor and add_offset local attributes of each SDS. The conversion equation then follows the HDF standard:
float_value = scale_factor * (integer_value – add_offset)
It is interesting to note that the add_offset local attribute is subtracted from (not added to) the stored integer. That is because the notation is reflective of how the data was packed (from the programmers perspective) rather than unpacked (from the data users perspective).